Data Mart vs Data Warehouse vs Database vs Data Lake
Not sure whether to invest in a data mart, data warehouse, database or data lake? Let's examine the key differences.
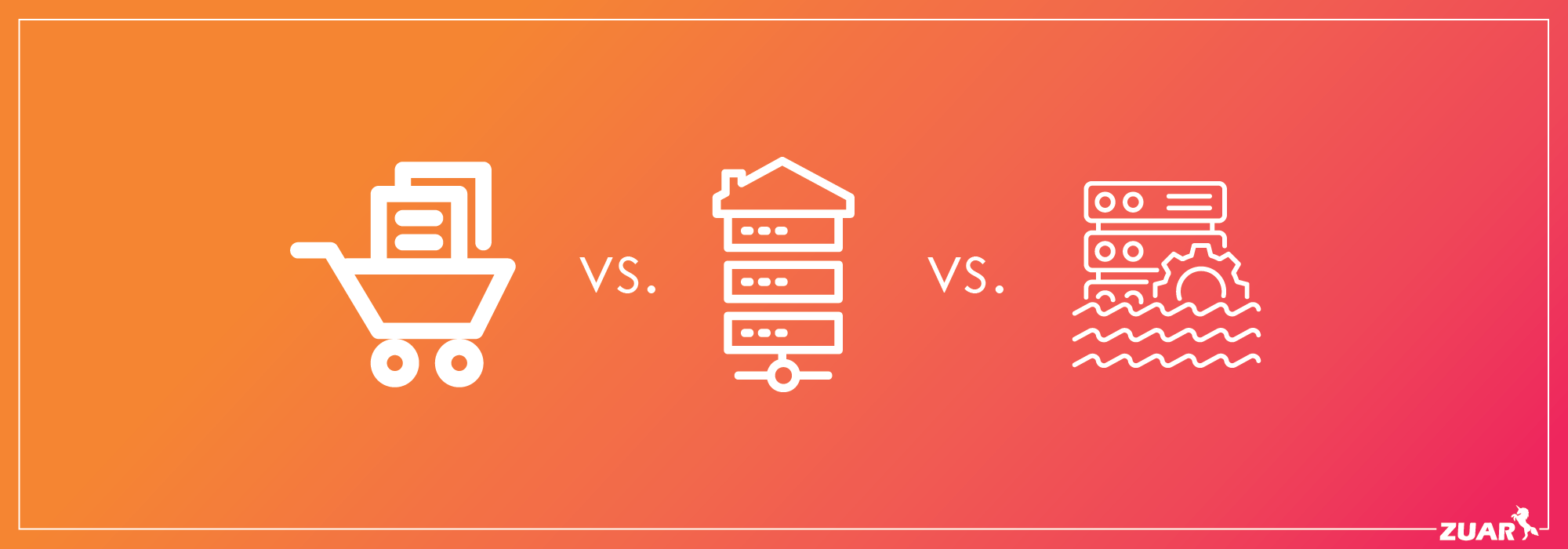
Every industry needs to process data. But the kind of data, scope, and use will illustrate if a data mart, data warehouse, database, or data lake will be the best solution for your enterprise.
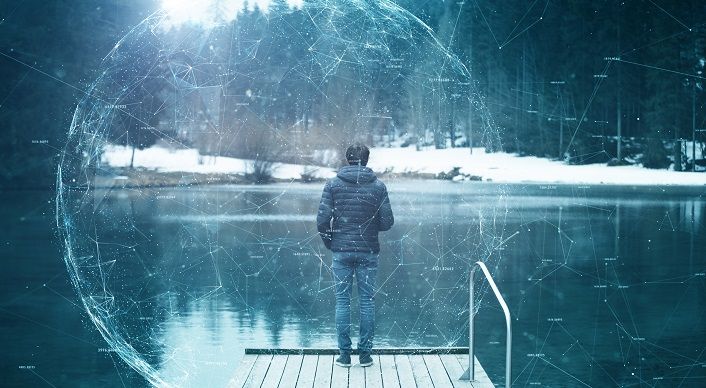
To get the best outcomes, it is critical that companies select the best enterprise data management system to fit their needs.
But which is better for your use cases? Is it more advantageous to use a data mart vs. a data warehouse? Or would it be better to utilize a data mart vs. data lake?
In this article, we'll illustrate the similarities and differences between these powerful technologies and outline the benefits and use cases they can bring to your organization.
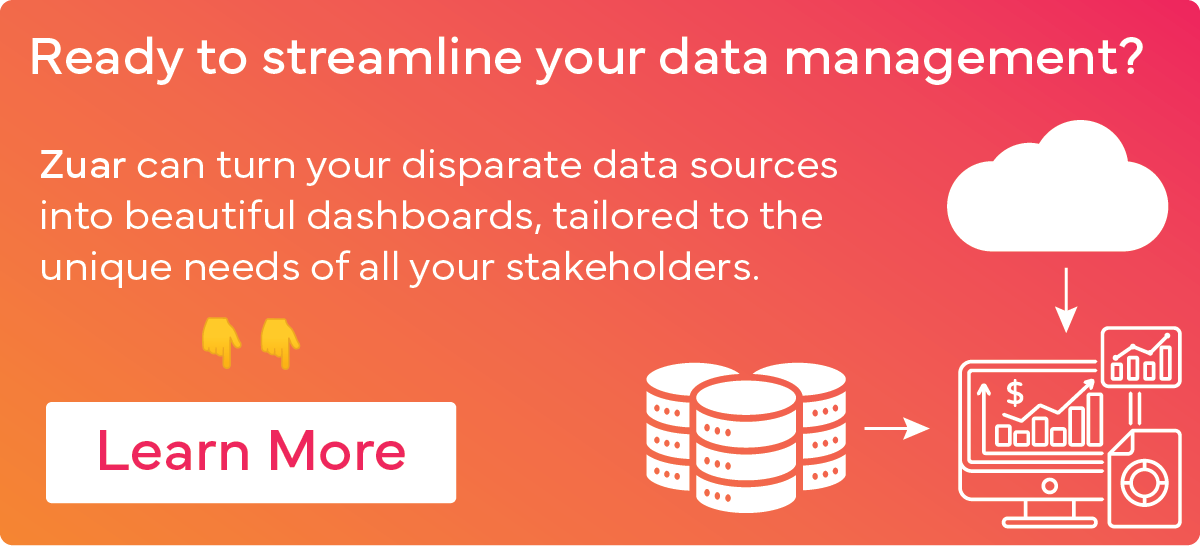
What is a Database?
A database is a storage location of related data used to capture a specific situation. One example of a database is a point-of-sale (POS) database. The POS database will capture and store all the relevant data surrounding a retail store’s transactions.
Databases have a variety of flavors: structured, relational, relational database management systems (RDBMS), or unstructured data structures (known as ‘NoSQL’). New data coming into the database is processed, organized, managed, updated and then stored in tables. MS Access is an example of an RDBMS while a platform like MongoDB is an example of a NoSQL database.
Databases are single-purpose repositories of raw transactional data. Because a database is closely tied with transactions, a database performs online transactional processing (OLTP).
Main Characteristics of Databases
- Structured according to company operations and applications
- Rigid rules set around data storage/organization (RDBMS-specific)
- Flexible data storage (NoSQL-specific)
- Single-purpose in its nature: handles one process (e.g., POS)
- Utilized for online transaction processing (OLTP)
- Data recording capabilities, capturing transactions as they occur and housing those transactions
What is a Data Warehouse?
A data warehouse is the core analytics system of an organization. The data warehouse will frequently work in conjunction with an operational data store (ODS) to ‘warehouse’ data captured by the various databases used by the business. For example, suppose a company has databases supporting POS, online activity, customer data, and HR data. In that case, the data warehouse will take the data from these sources and make them available in a single location. Again, the ODS will typically handle the process of cleaning and normalizing the data, preparing it for storage in the data warehouse.
The method of extracting data from the database, transforming it in the ODS, and loading it into the data warehouse is an example of the extract-transform-load (ETL) process, or the similar ELT process.
Because a data warehouse captures transformed (i.e. cleaned) historical data, it is an ideal tool for data analysis. Because business units will leverage the warehouse data to create reports and perform data analysis, business units are frequently involved in how the data is organized. Like a relational database, it typically uses SQL to query the data, and it uses tables, indexes, keys, views, and data types for data organization and integrity.
While a database can be a pseudo-data warehouse through the implementation of views, it is considered best practice to use a data warehouse for business user interaction leaving databases to capture transactional data. Because the chief intent is analytics, a data warehouse is used for online analytical processing (OLAP). OLAP is actually Zuar’s bread and butter, with our Zuar Runner solution making it possible for companies to automate their ETL/ELT processes.
Main Characteristics of a Data Warehouse
- Stores large quantities of historical data so old data is not erased when new data is updated
- Captures data from multiple, disparate databases
- Works with ODS to house normalized, cleaned data
- Organized by subject
- OLAP (online analytical processing) application
- The primary data source for data analytics
- Reports and dashboards use data from data warehouses
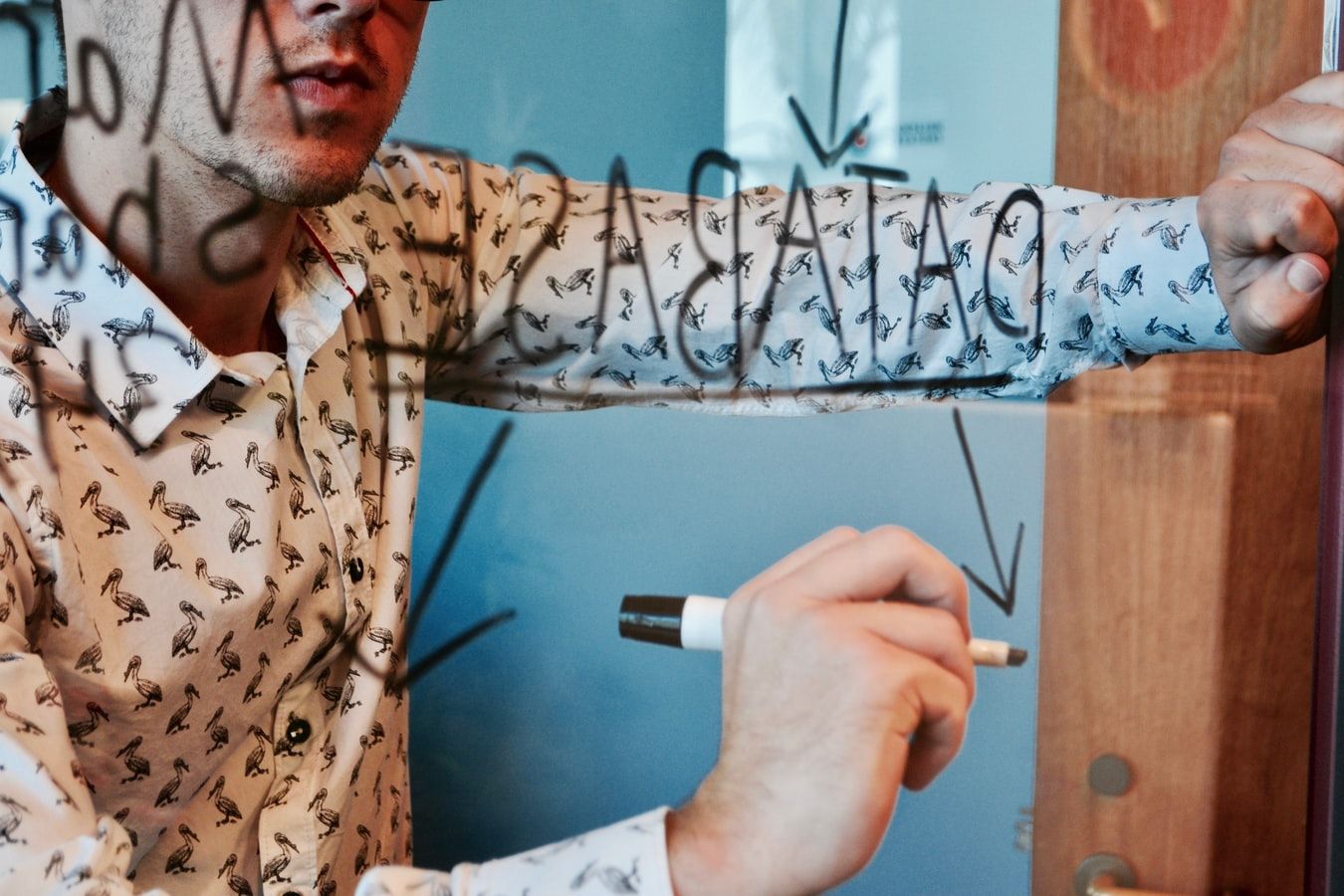
What is a Data Mart?
A data mart is very similar to a data warehouse. Like a data warehouse, the data mart will maintain and house cleaned data ready for analysis. However, unlike a data warehouse, the scope of visibility is limited.
A data mart supplies subject-oriented data necessary to support a specific business unit. For example, a data mart could be created to support reporting and analysis for the marketing department. By limiting the data to a particular business unit (for example, the marketing department), the business unit does not have to sift through irrelevant data.
Another benefit is security. Limiting the visibility of non-essential data to the department eliminates the chance of that data being used irresponsibly.
A third benefit is speed. As there will be less data in the data mart, the processing overhead is decreased. This means that queries will run faster.
Finally, because the data in the data mart is aggregated and prepared for that department appropriately, the chance of misusing the data is reduced. The potential for conflicting reporting is also reduced.
Main Characteristics of a Data Mart
- Focuses on one subject matter or business unit
- Acts as a mini-data warehouse, holding aggregated data
- Data is limited in scope
- Often uses a star schema or similar structure
- Reports and dashboards use the data from the data mart
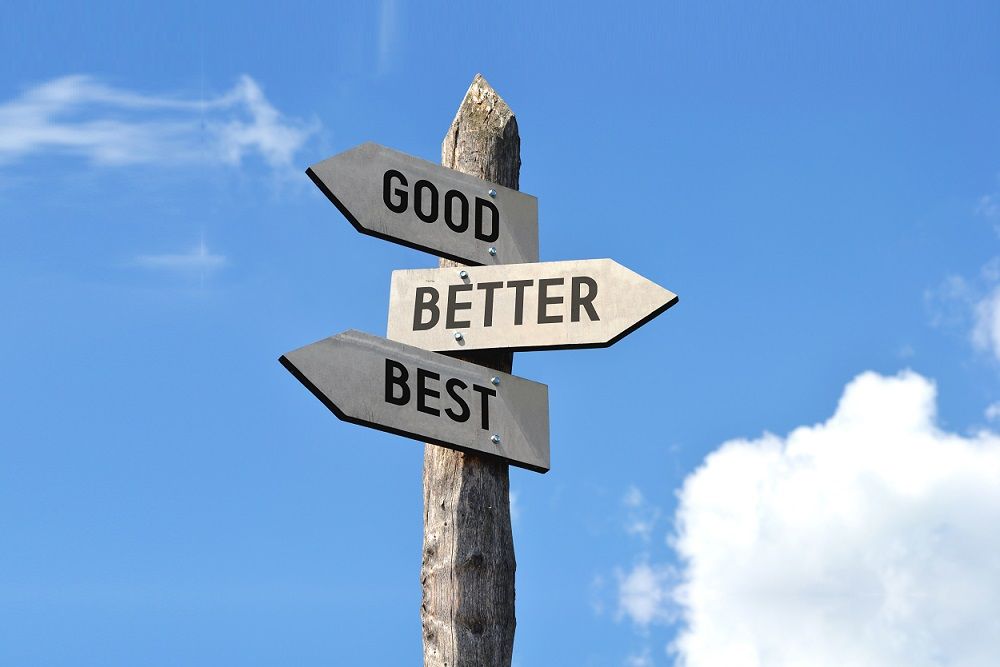
What is a Data Lake?
A data lake stores an organization’s raw and processed (unstructured and structured) data at both large and small scales. Unlike a data warehouse or database, a data lake captures anything the organization deems valuable for future use. This can be images, videos, PDFs, anything! The data lake will extract data from multiple disparate data sources and process the data like a data warehouse. Also, like a data warehouse, a data lake can be used for data analytics and report creation. However, the technology used in a data lake is much more complex than in a data warehouse.
Different applications and technologies, such as Java, are used for its processing and analysis. Frequently, data lakes are used in conjunction with machine learning. The output from machine learning tests is also often stored as well in the data lake. Because of the level of complexity and skill required to leverage, a data lake requires users who are experienced in programming languages and data science techniques. Lastly, unlike a data warehouse, a data lake does not leverage an ODS for data cleaning.
Main Characteristics of a Data Lake
- Collects all data from many disparate data sources over an extended period
- Meets the needs of various users in the organization
- It is uploaded without an established methodology
- Processes and cleans data and stores it in the data lake
How Are Data Lakes Utilized?
A data lake is an excellent complementary tool to a data warehouse because it provides more query options. A data warehouse will provide structured and organized information. However, with the addition of a data lake, the organization can tap into raw data that may offer even more insight or support because data lakes provide real-time analytics.
Data marts and data lakes create two sides of the spectrum, where data marts are focused data, and data lakes are enormous repositories of raw data.
The research and science fields depend heavily on data lake architecture.. Data lakes are suitable for scientific use because not only is the data raw from feedback sources and algorithms; it’s also real-time. Science is only as good as its most current and relevant deductions. Research needs to be fresh to have an impact on the reports or findings that it produces.
In enterprise, data marts are mainly used internally for department-based information. Since it’s condensed and summarized, data mart information derived from the broader data warehouse allows each department to access more focused data to its operations.
Data Lake Architecture
This model provides a typical use of a data lake. The data lake represents an all-in-one process. The data lake represents an all-in-one process. Data comes from disparate sources (databases, various raw data from images, etc.). The data lake process is circular. The ETL process is performed in the data lake, and the cleaned data is then stored inside the data lake. The cleaned data sets become the source for reports and dashboards.
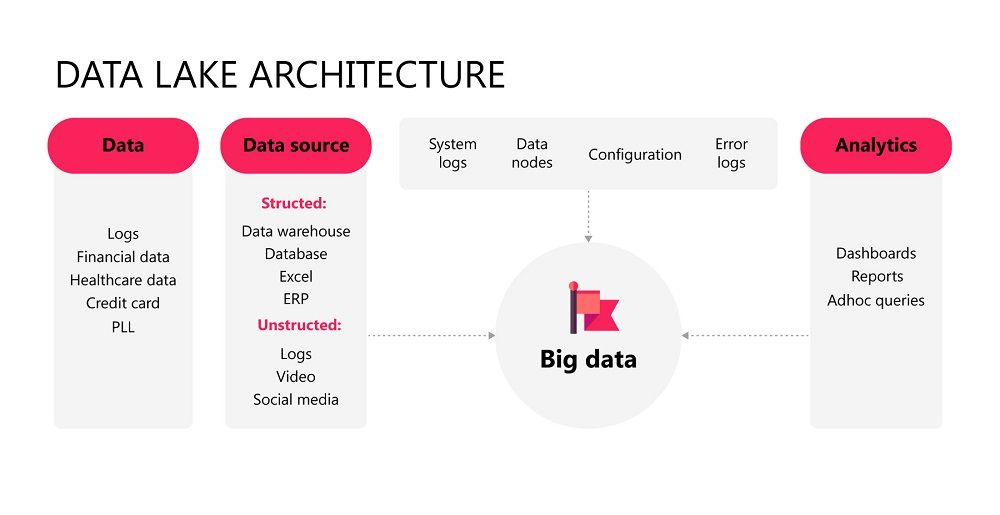
Database, Data Warehouse & Data Mart Architecture
This model provides a view of how the database, data warehouse, and data mart work together. The databases each represent a single transactional source. An ETL process is performed, preparing the data to send to the operational data store (ODS). The ODS processes the data for the data warehouse. From the data warehouse, subject-specific, limited data sets are fed to the various data marts. Finally, from the data marts, reports and dashboards are created. While the diagram does not show it, reports and dashboards can be made directly from the data warehouse as well.
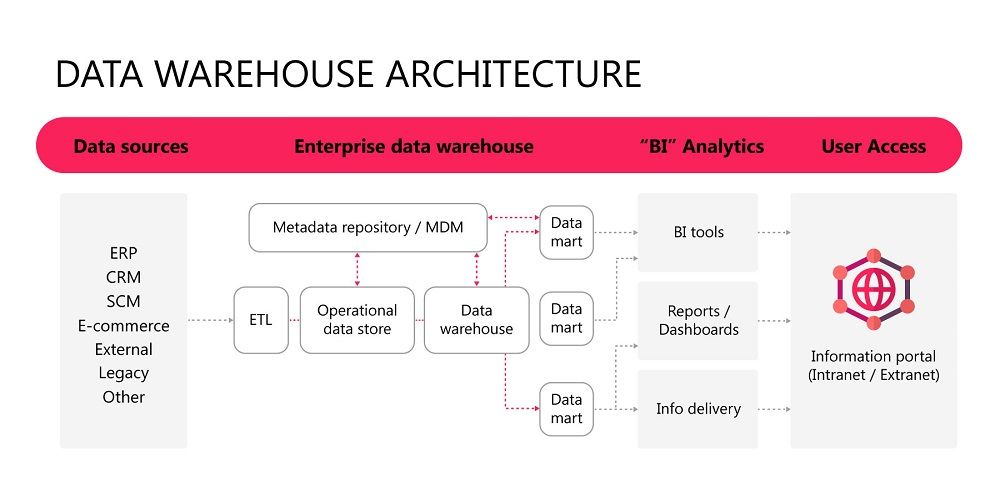
Data Warehouse vs. Databases
The main difference between these two include:
- Data warehouses store summarized data while databases utilize detailed data.
- Databases capture transactions, unlike data warehouses, which are used to analyze data.
- Databases house current information but the warehouses house both historical and current information.
- Databases capture data from one primary source, while data warehouses provide information from various sources.
- In conjunction with reporting and analytics tools, a data warehouse provides insight into the company’s overall business operations while a database captures fundamental day-to-day operations.
Data Mart vs. Data Warehouse
The key differences between a data mart vs. a data warehouse include:
- Data marts are smaller, subject-specific subsets of data extracted from a data warehouse.
- Data marts are a repository of essential data for a specific subgroup. Only a few users have access to the entire data warehouse.
- Data marts require less overhead and can analyze data faster because they are smaller subsets of the data warehouse.
- A data warehouse is significantly larger, generally a terabyte or more in size, where a data mart is usually less than 100 GB.
- Data warehouses contain all the cleaned, normalized data across the business units of an organization where a data mart has a smaller scope, typically focused on one line of business.
- A data warehouse gets its data from databases; a data mart gets its data from the data warehouse.
Data Lake vs. Data Mart
The key differences between a data lake vs. a data mart include:
- Data lakes contain all the raw, unfiltered data from an enterprise where a data mart is a small subset of filtered, structured essential data for a department or function.
- Data marts are very specific, allowing for fast, effective analytics of relevant summarized information. Data lakes are better for broader, deep analysis of raw data.
- Data lakes are more an all-in-one solution, acting as a data warehouse, database, and data mart. A data mart is a single-use solution and does not perform any data ETL.
- Data lakes have a central archive where data marts can be stored in different user areas.
Data Warehouse vs. Data Lake
The key differences between a data warehouse vs. a data lake include:
- A data lake stores all the data for the organization. A data warehouse will store cleaned data for creating structured data models and reporting.
- Data lakes utilize different hardware that allows for cost-effective terabyte and petabyte storage.
- Data warehouses typically use an ODS from transactional systems. A data lake will extract data from all data types, including non-traditional data types like web server logs, social network activity, sensor data, etc.
- Data warehouses are for operational users that need to generate reports for analytics. A data lake is for deep analysis that goes beyond the stored data of a data warehouse.
- Because data lakes store raw data that can be accessed and searched before it has been cleansed or structured, a user can retrieve results faster. However, this is dependent upon the skill set of the user.
Database vs. Data Mart
The key differences between a database vs. a data mart include:
- A database is a transactional data repository (OLTP). A data mart is an analytical data repository (OLAP).
- A database captures all the aspects and activities of one subject in particular. A data mart will house data from multiple subjects.
- The data in a database will be raw and unprocessed (not cleaned). The data in a data mart will be processed and validated for greater reporting ease.
- Users do not interact with data in a database. Users directly interact with data from a data mart.
- Databases are the first step in the data ETL process. Data marts are the last step in the ETL process.
Database vs. Data Lake
The key differences between a database vs. a data lake include:
- A database captures transactional data associated with one topic or subject. A data lake captures activity from many databases and other disparate data sources.
- A database stores traditional data, such as text and numeric data. A data lake can capture any type of data, such as PDFs, image files, sound files, etc.
- A database does not do any data cleansing. It stores the raw, unprocessed data. While a data lake will also store raw data, it will also implement data cleaning procedures.
- A database will export its data to another process (the operational data store or ODS). The data lake will do all the data processing (cleaning, aggregation) internally.
- Databases are the first step in the data ETL process. A data lake handles all aspects of the ETL process.
Database, Data Warehouse vs. Data Lake
The key differences between the combination of database and data warehouse vs. a data lake include:
- Multiple databases connect to a data warehouse via an external tool, such as an operational data store (ODS). The data lake does not require an ODS.
- An ODS is used between databases, and the data warehouse will perform the analytical processing and data cleaning. The data lake will perform all analysis and data cleaning ‘in-house.’
- The database and data warehouse will often supply more refined data to a data mart. The data lake does not require a data mart. The data lake feeds refined data directly to reports, dashboards, etc.
Database, Data Warehouse, Data Mart vs. Data Lake
The key differences between the combination of database, data warehouse, and data mart vs. a data lake include:
- A data lake performs all the operations as the amalgam of database(s), data warehouse, and data mart (in conjunction with the ODS).
- A data lake does not utilize an ODS. The database(s) leverages an ODS to transform the data and load it into the data warehouse.
- A data lake requires greater programming skills to use. The database(s), data warehouse, and data mart use SQL and less code-heavy skillsets.
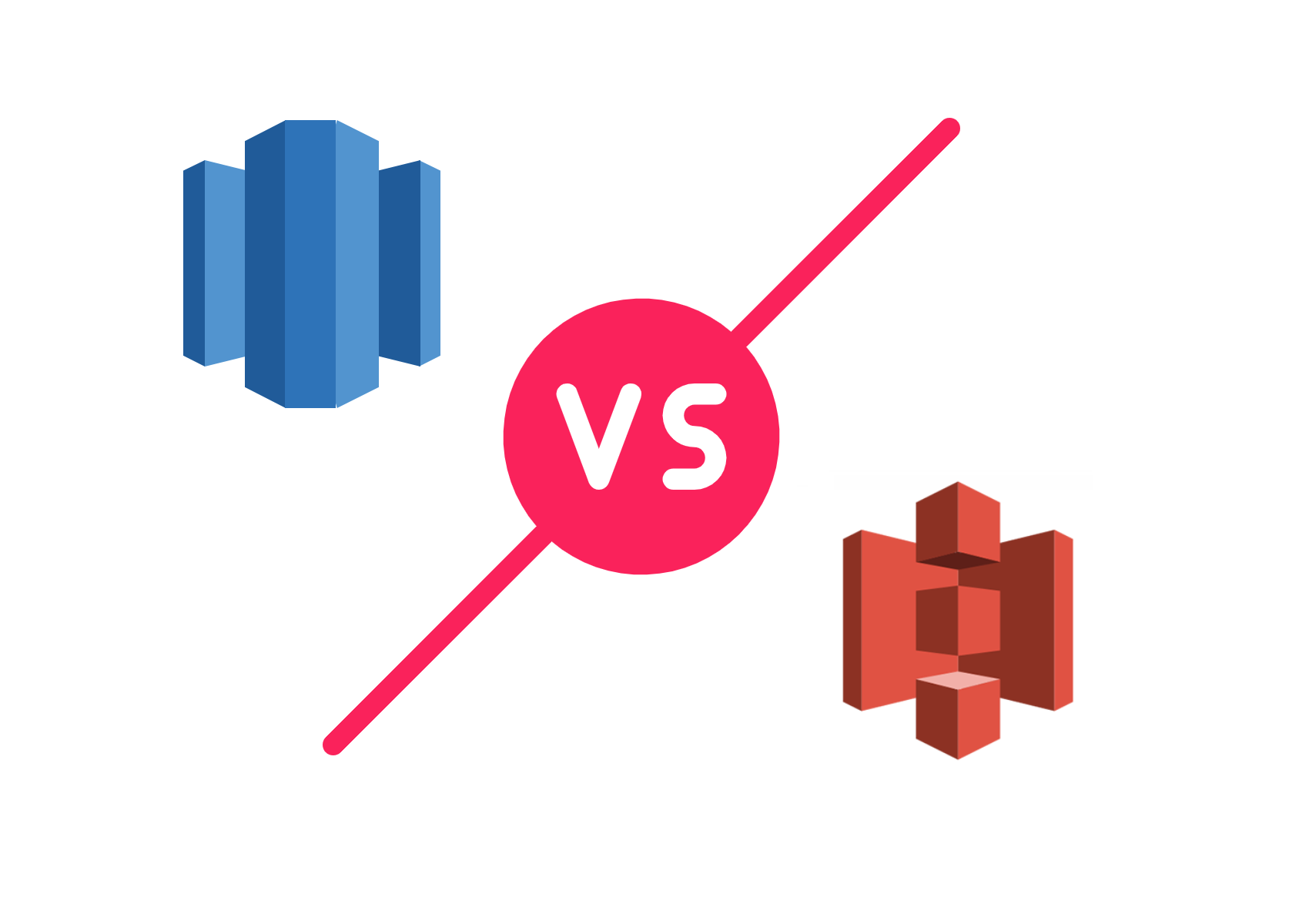
Expert Help
This stuff is complex. But that's why Zuar was founded. We work with organizations of all sizes to help them get set up with data pipelines that utilize up-to-date yet proven technologies.
Additionally, when it comes to managing and leveraging your data, having the right tools is crucial. Zuar Runner offers a powerful data pipeline solution, while Zuar Portal takes it a step further by providing a centralized hub for data collaboration and governance.
With Zuar Portal, you can easily manage data access, track data lineage, and collaborate with team members, all in one place. Seamlessly integrate Zuar Portal with Zuar Runner for a comprehensive and efficient data management ecosystem.
Make informed decisions, drive innovation, and propel your business forward with Zuar Runner and Zuar Portal. Try it today and revolutionize the way you handle your data.
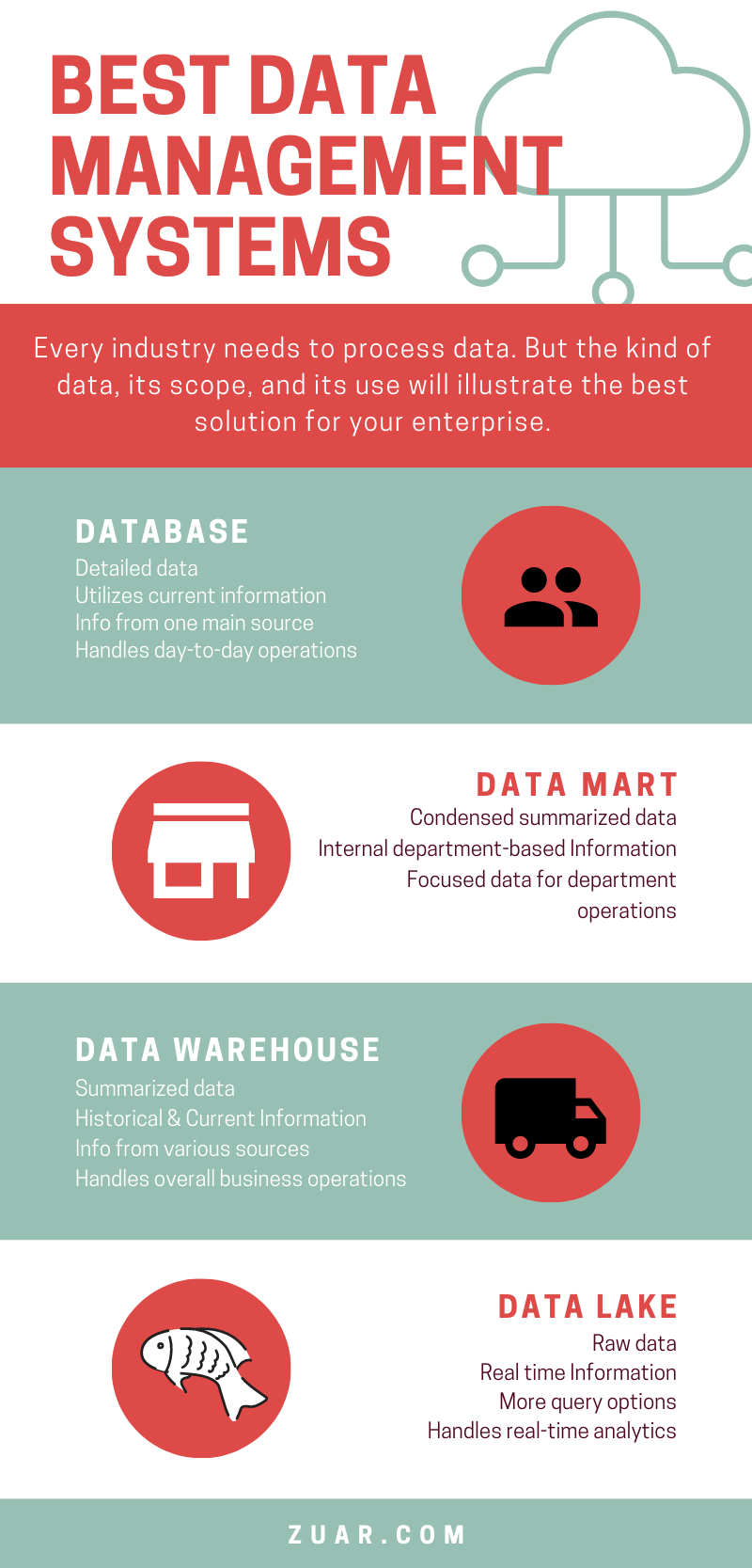
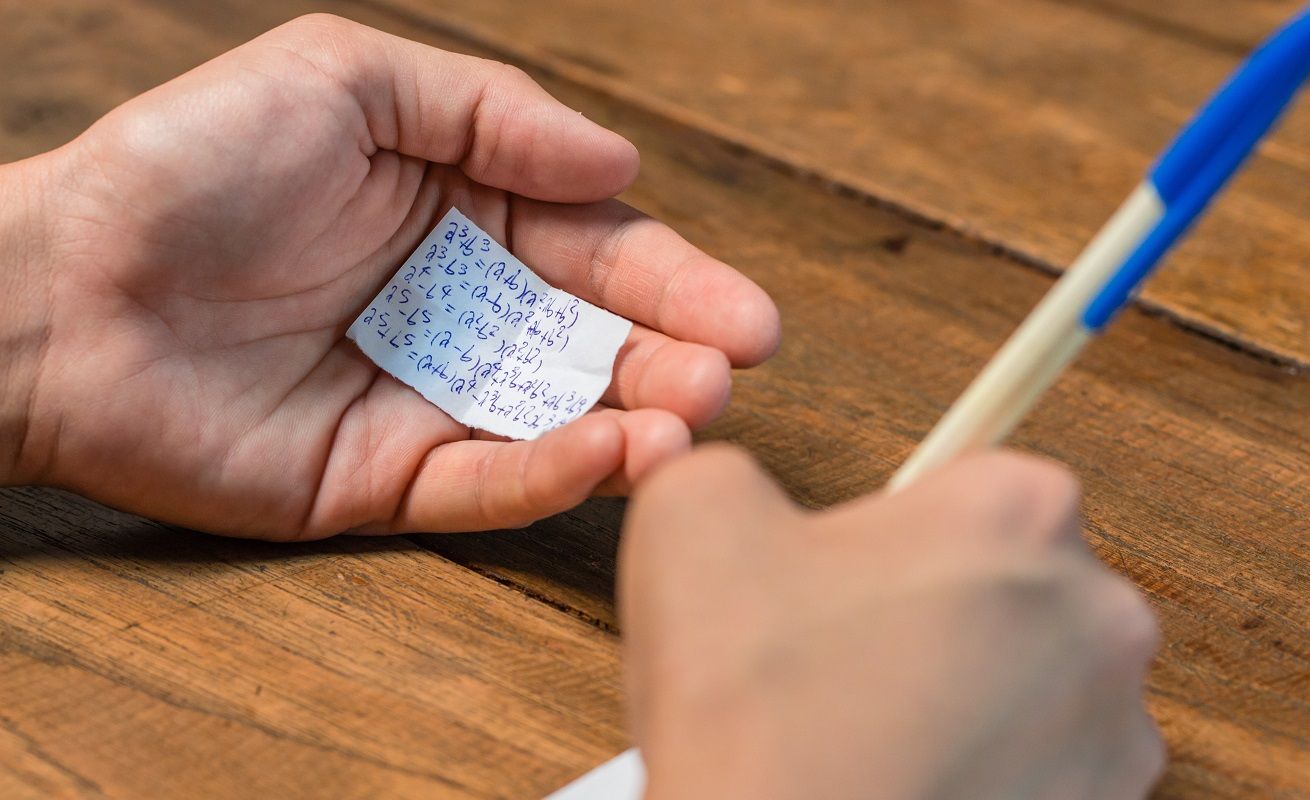
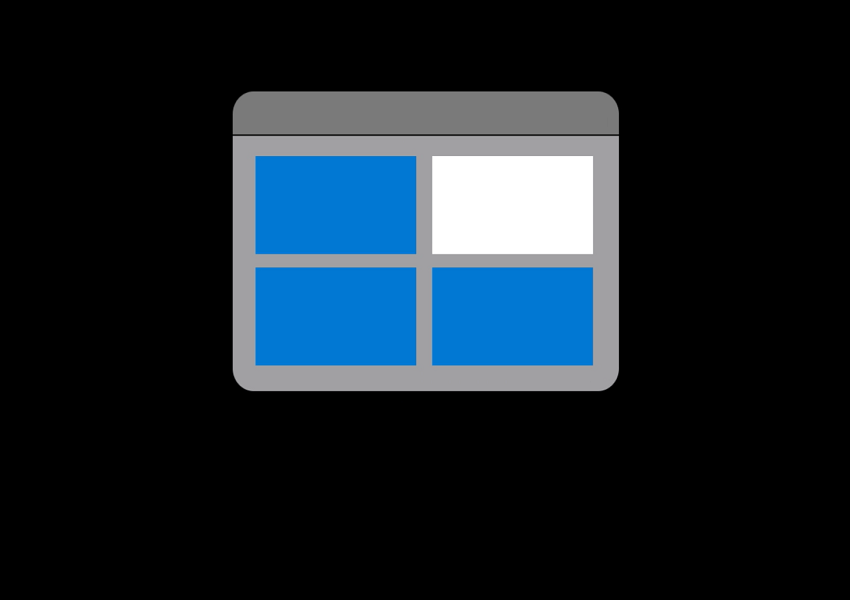