Data Transformation: Types, Process, Benefits & Definition
What is data transformation? What are the benefits of data transformation? What are the processes? Learn all this and more!
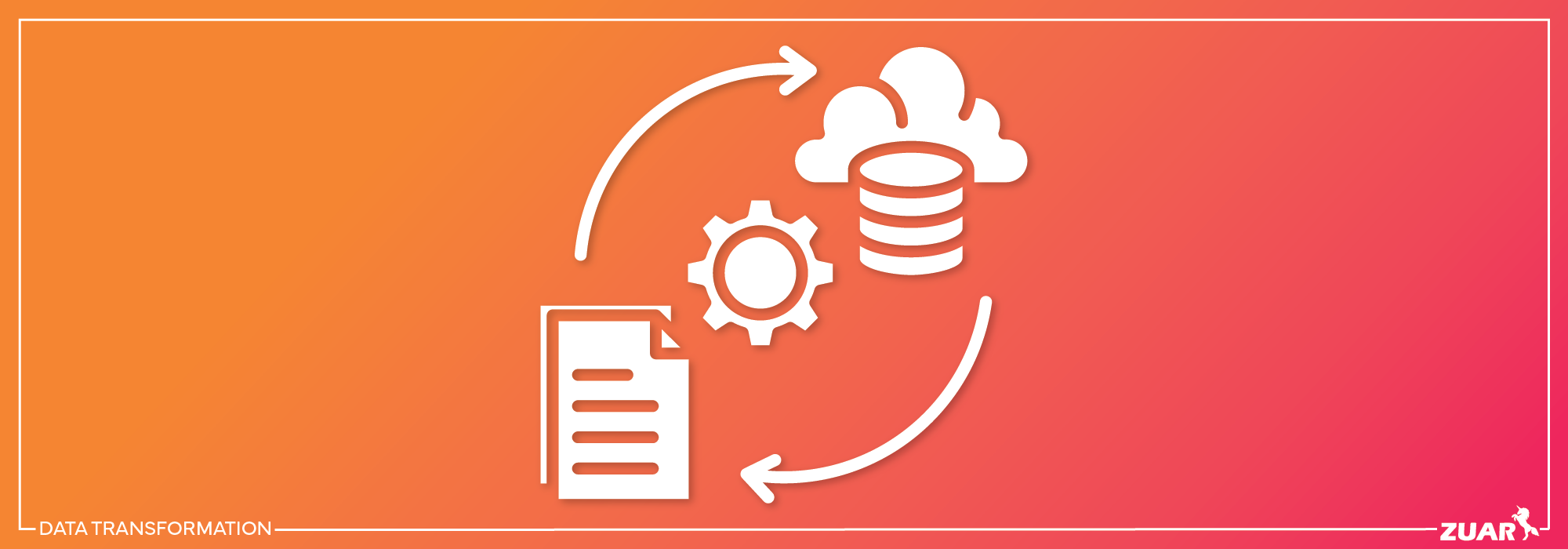
What Is Data Transformation?
Data transformation refers to the process of converting, cleaning, and manipulating raw data into a structured format that is suitable for analysis or other data processing tasks.
The last few decades have seen a renaissance in data collection and processing—today’s data teams have more information at their disposal than ever before.
While this has led to a proliferation of data analytics and science, it’s presented a number of problems for engineers and business teams.
Raw data can be challenging to work with and difficult to filter. Often, the problem isn’t how to collect more data, but which data to store and analyze.
To curate appropriate, meaningful data and make it usable across multiple systems, businesses must leverage data transformation.
Key Takeaways
- Data transformation is the process of converting raw data into a structured, standardized format to enable better analysis and decision-making.
- Key steps in data transformation include discovering data, mapping modifications, extracting data, executing code to transform it, reviewing for correctness, and loading the output.
- Benefits of data transformation include improved data quality, speed, organization, and management, but it can be expensive and requires contextual awareness to avoid errors.

Understanding Data Transformation
Raw data can be challenging to work with and difficult to filter. Often, the problem isn’t how to collect more data, but which data to store and analyze.
Transformation may occur on the format, structure, or values of data. With regard to data analytics, transformation usually occurs after data is extracted or loaded (ETL/ELT).
Data transformation increases the efficiency of analytic processes and enables data-driven decisions. Raw data is often difficult to analyze and too vast in quantity to derive meaningful insight, hence the need for clean, usable data.
During the transformation process, an analyst or engineer will determine the data structure. The most common types of data transformation are:
- Constructive: The data transformation process adds, copies, or replicates data.
- Destructive: The system deletes fields or records.
- Aesthetic: The transformation standardizes the data to meet requirements or parameters.
- Structural: The database is reorganized by renaming, moving, or combining columns.
In addition, a practitioner might also perform data mapping and store data within the appropriate database technology.
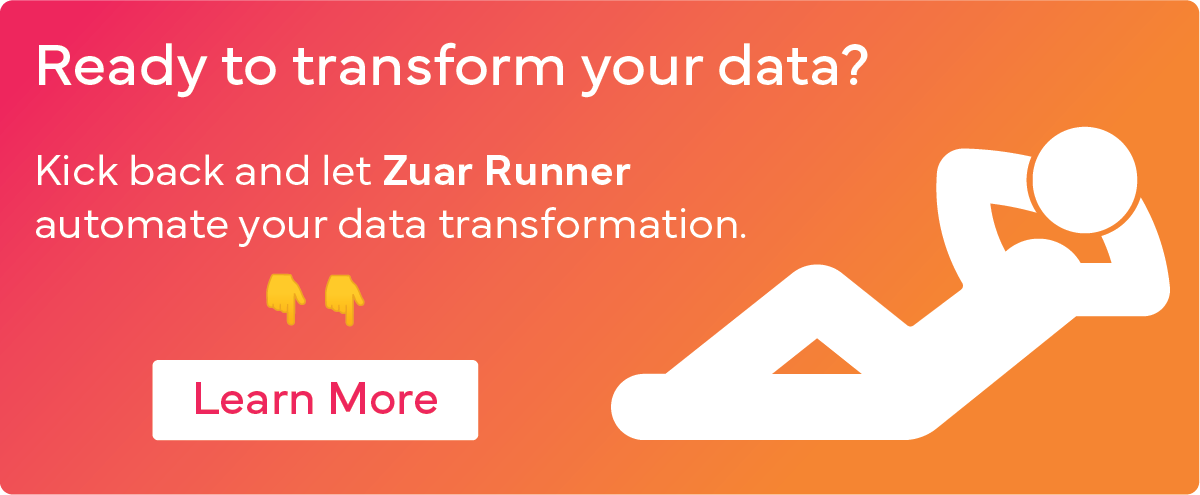
The Data Transformation Process
In a cloud data warehouse, the data transformation process most typically takes the form of ELT (Extract Load Transform) or ETL (Extract Transform Load). With cloud storage costs becoming cheaper by the year, many teams opt for ELT— the difference being that all data is loaded in cloud storage, then transformed and added to a warehouse.
The transformation process generally follows 6 stages:
- Data Discovery: During the first stage, data teams work to understand and identify applicable raw data. By profiling data, analysts/engineers can better understand the transformations that need to occur.
- Data Mapping: During this phase, analysts determine how individual fields are modified, matched, filtered, joined, and aggregated.
- Data Extraction: During this phase, data is moved from a source system to a target system. Extraction may include structured data (databases) or unstructured data (event streaming, log files) sources.
- Code Generation and Execution: Once extracted and loaded, transformation needs to occur on the raw data to store it in a format appropriate for BI and analytic use. This is frequently accomplished by analytics engineers, who write SQL/Python to programmatically transform data. This code is executed daily/hourly to provide timely and appropriate analytic data.
- Review: Once implemented, code needs to be reviewed and checked to ensure a correct and appropriate implementation.
- Sending: The final step involves sending data to its target destination. The target might be a data warehouse or other database in a structured format.
These steps are meant to illustrate patterns of data transformation— no single “correct” transformation process exists. The right process is the one that works for your data team. That is to say, other bespoke operations might occur in a transformation.
For example, analysts may filter data by loading certain columns. Alternatively, they might enrich the data with names, geo-properties, etc. or dedupe and join data from multiple sources.
Related Article:
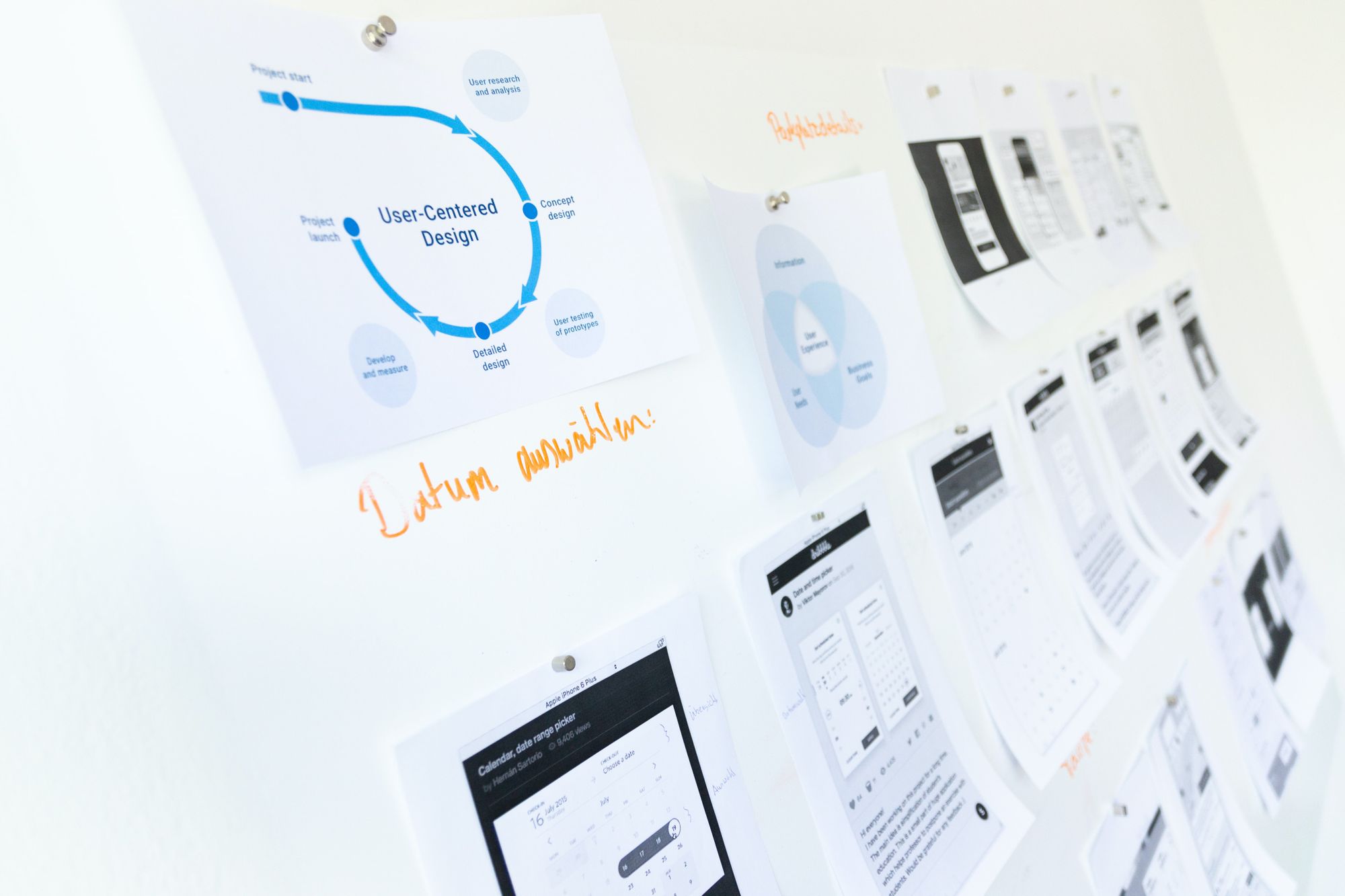

Data Transformation Types
There are two common approaches to data transformation in the cloud: scripting-/code-based tools and low-/no-code tools. Scripting tools are the de-facto standard, with the greatest amount of customization, flexibility, and control over how data is transformed. Nonetheless, low-code solutions have come a long way, specifically in the last few years. We’ll briefly discuss both options.
Scripting Tools
The most common data transformations occur using SQL or Python. At the simplest, these transformations might be stored in a repository and executed using some orchestrator. More commonly, platforms like dbt are used to orchestrate and order transformations using a combination of SQL/Python. These tools or systems often boil down to programmatically creating tables or transformations using some scripting language.
The Python Runner SDK is also useful for scripting and automation. Enabling remote interactions with schedules, jobs, and business functions has never been easier. Want to see Runner in action? Schedule a demo of the Python Runner SDK.
Low-/No-Code Tools
These data transformation tools are the easiest for non-technical users to utilize. They allow you to collect data from any cloud source and load it into your data warehouse using an interactive GUI. Over the past decade, many low-code solutions have proliferated.
Zuar Runner is an example of a product that has ETL/ELT capabilities, but also helps you manage data at every step in its journey. Runner can be hosted either on-premise or in the cloud and has code and no code options.
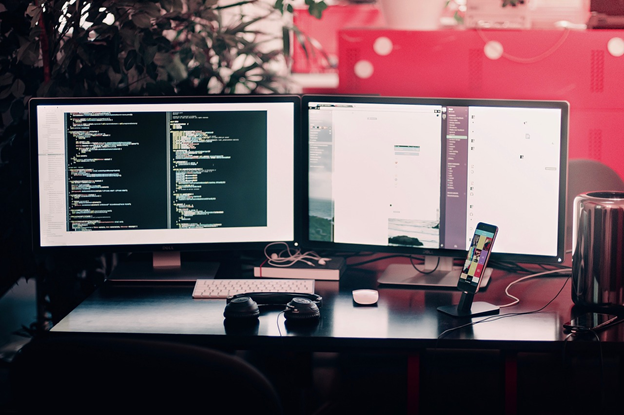
Data Transformation Techniques
There are several data transformation techniques that can help structure and clean up the data before analysis or storage in a data warehouse. Here are some of the more common methods:
- Smoothing: This is the data transformation process of removing distorted or meaningless data from the dataset. It also detects minor modifications to the data to identify specific patterns or trends.
- Aggregation: Data aggregation collects raw data from multiple sources and stores it in a single format for accurate analysis and reports. This technique is necessary when your business collects high volumes of data.
- Discretization: This data transformation technique creates interval labels in continuous data to improve efficiency and easier analysis. The process utilizes decision tree algorithms to transform a large dataset into compact categorical data.
- Generalization: Utilizing concept hierarchies, generalization converts low-level attributes to high-level, creating a clear data snapshot.
- Attribute Construction: This technique allows a dataset to be organized by creating new attributes from an existing set.
- Normalization: Normalization transforms the data so that the attributes stay within a specified range for more efficient extraction and data mining applications.
- Manipulation: Manipulation is the process of changing or altering data to make it more readable and organized. Data manipulation tools help identify patterns in the data and transform it into a usable form to generate insight.

Data Transformation: Benefits and Challenges
Data transformation offers several benefits and challenges in the realm of data management.
Data Transformation Benefits
Transforming data can help businesses in a variety of ways. Here are some of the biggest benefits:
- Better Organization: Transformed data is easier for both humans and computers to use. The process of transformation involves assessing and altering data to optimize storage and discoverability.
- Improved Data Quality: Bad data poses a number of risks. Data transformation can help your organization eliminate quality issues and reduce the possibility of misinterpretation.
- Faster Queries: By standardizing data and storing it properly in a warehouse, query speed and BI tooling can be optimized— resulting in lower friction to analysis.
- Simpler Data Management: A large part of data transformation is metadata and lineage tracking. By implementing these techniques, teams can drastically simplify data management. This is especially important as organizations grow and demand data from a large number of sources.
- Broader Use: Transformation makes it easier to get the most out of your data by standardizing and making it more usable.
Data Transformation Challenges
While the methods of data transformation come with numerous benefits, it’s important to understand that a few potential drawbacks exist.
- Transformation can be expensive and resource-intensive: While processing and compute costs have fallen in recent years, it’s not uncommon to hear stories of extreme AWS, GCP, or Databricks bills. Furthermore, the resource cost from a man-hour/salary perspective is hefty: most companies require a team of data analysts/engineers/scientists to extract value from data.
- Contextual awareness is crucial: If analysts/engineers transforming data lack business context or understanding, extreme errors are possible. While data observability tooling continues to improve, there are some errors that are almost undetectable and could lead to misinterpreting data or making an incorrect business decision.
Nonetheless, data transformation is an essential part of any data-driven organization. Implementing tests and following the best-practices of software development will help to minimize errors and improve confidence in data.
Without experienced data analysts with the right subject matter expertise, problems may occur during the data transformation process. While the benefits of data transformation outweigh the drawbacks, it's necessary to take appropriate caution to ensure sound transformation.

Data Transformation Implementation
Organizing, transforming, and structuring data can be an overwhelming task for many organizations, but with the right research and planning it's possible to integrate a data-driven culture into your business.
And that's where Zuar Runner shines. With its end-to-end ELT solution, Zuar Runner eliminates the need for connecting multiple pipeline tools, saving both time and money.
By automating workflows, from raw data extraction to end-user visualization, data teams can reclaim their time and focus on implementing advanced analytics.
Here's a case study for Manduka, a retail company offering yoga equipment and apparel:
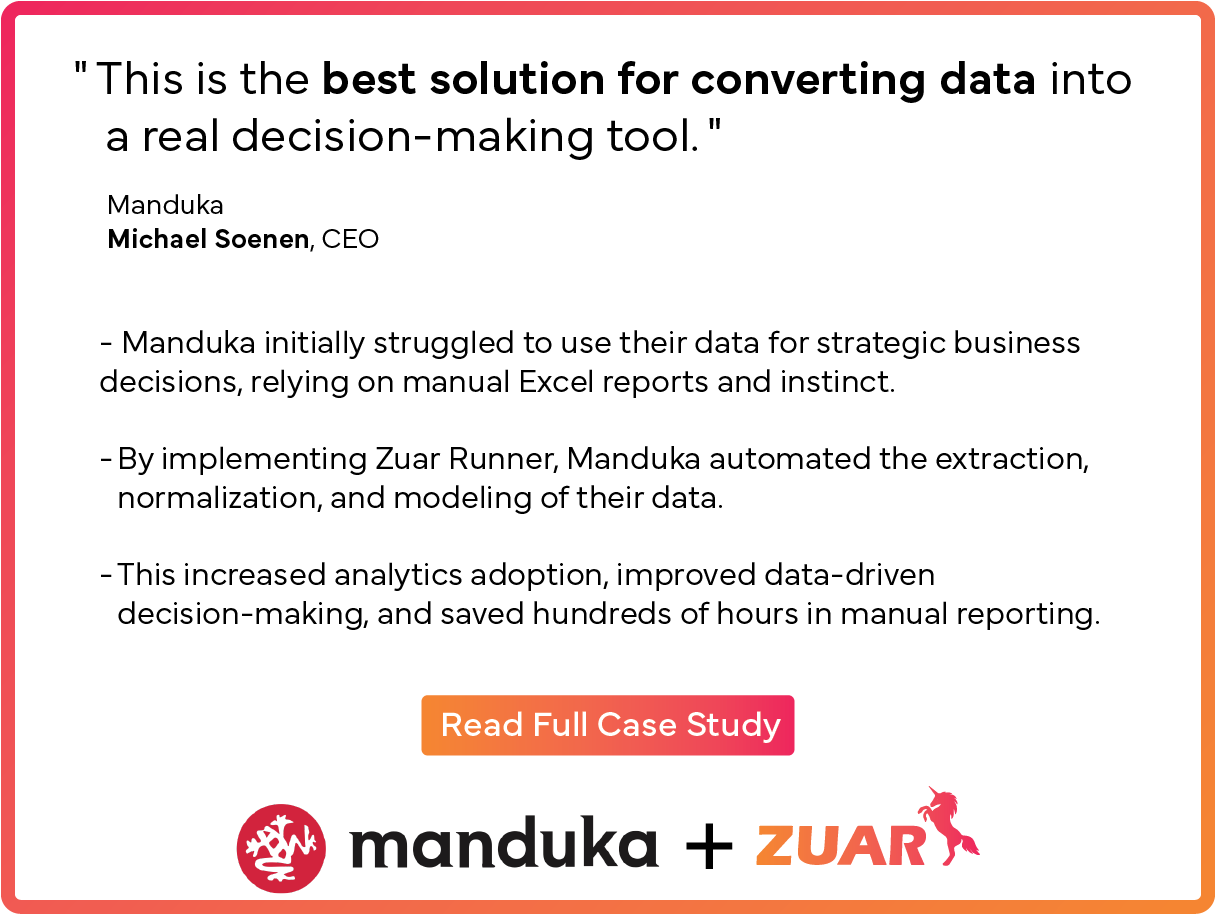
Zuar Runner's pre-built connectors and flexibility to add custom data sources provide effortless connectivity to almost any data format. With Zuar Runner, data transformation becomes a seamless and cost-effective process, enabling organizations to deploy enterprise-level tools without the hefty price tag.
Stop wasting time on manual data transformation and embrace the power of Zuar Runner to revolutionize your data pipeline and unlock the true potential of your data. Talk with one of our data experts to learn more and set up a demo!
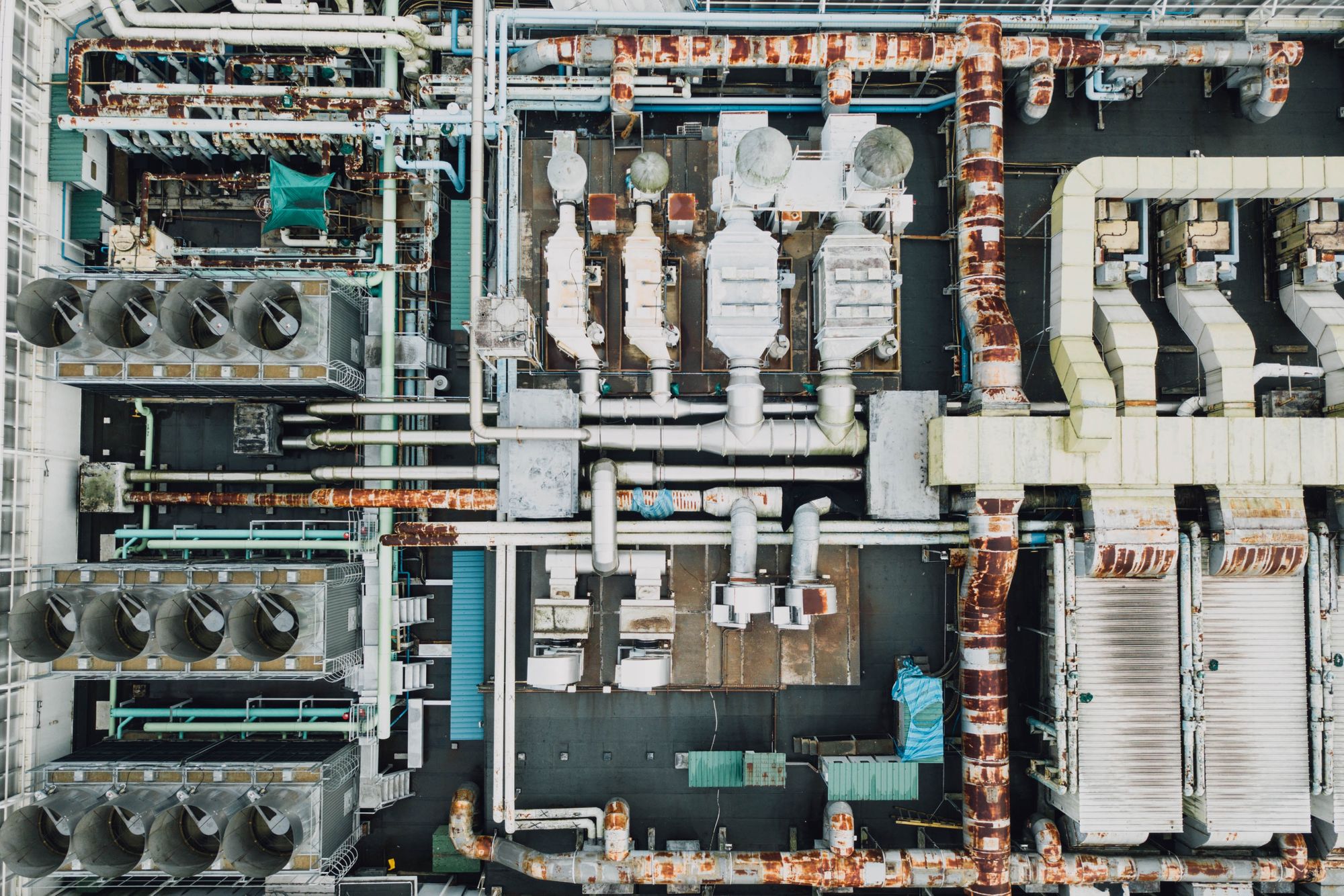
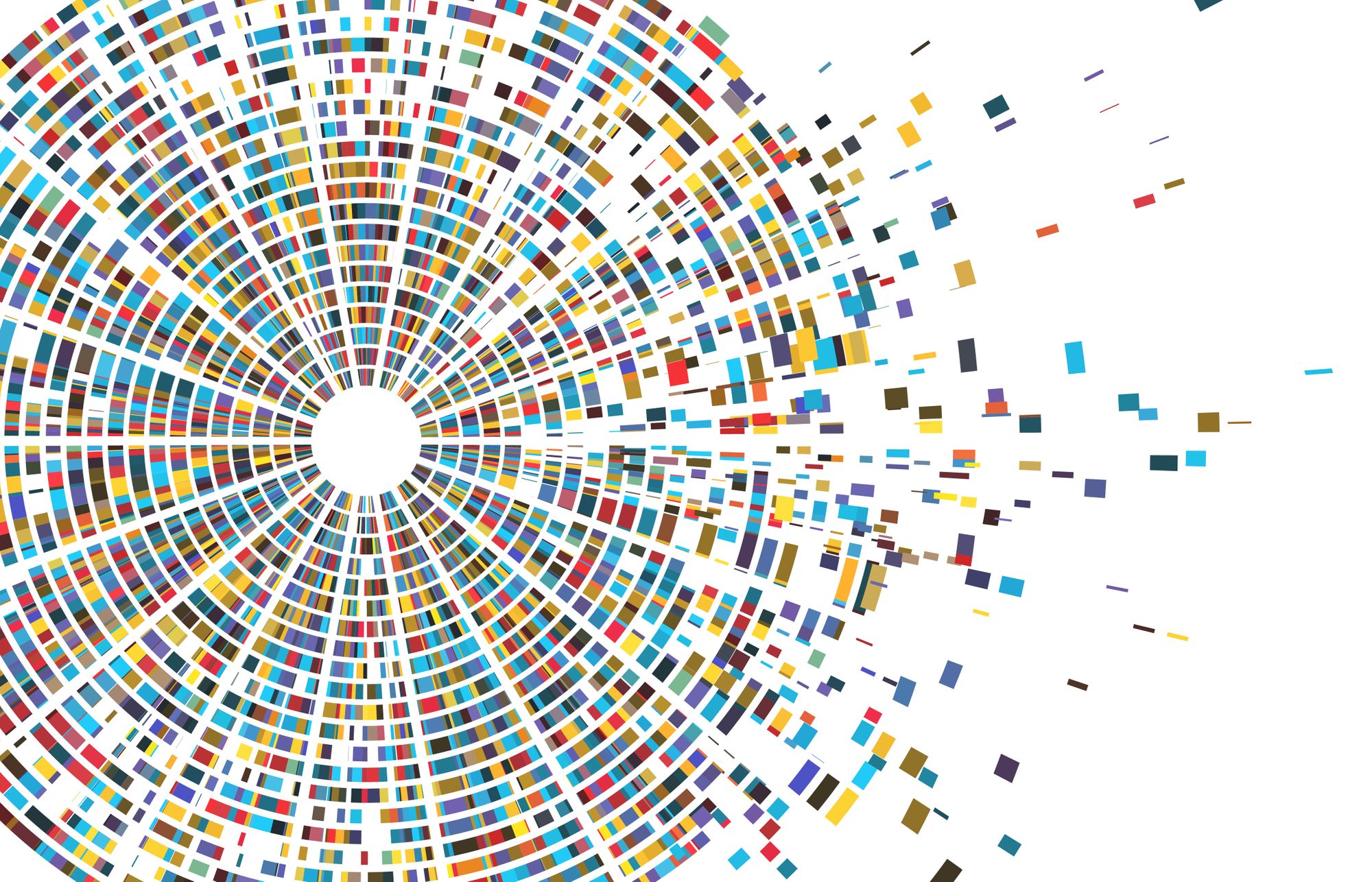
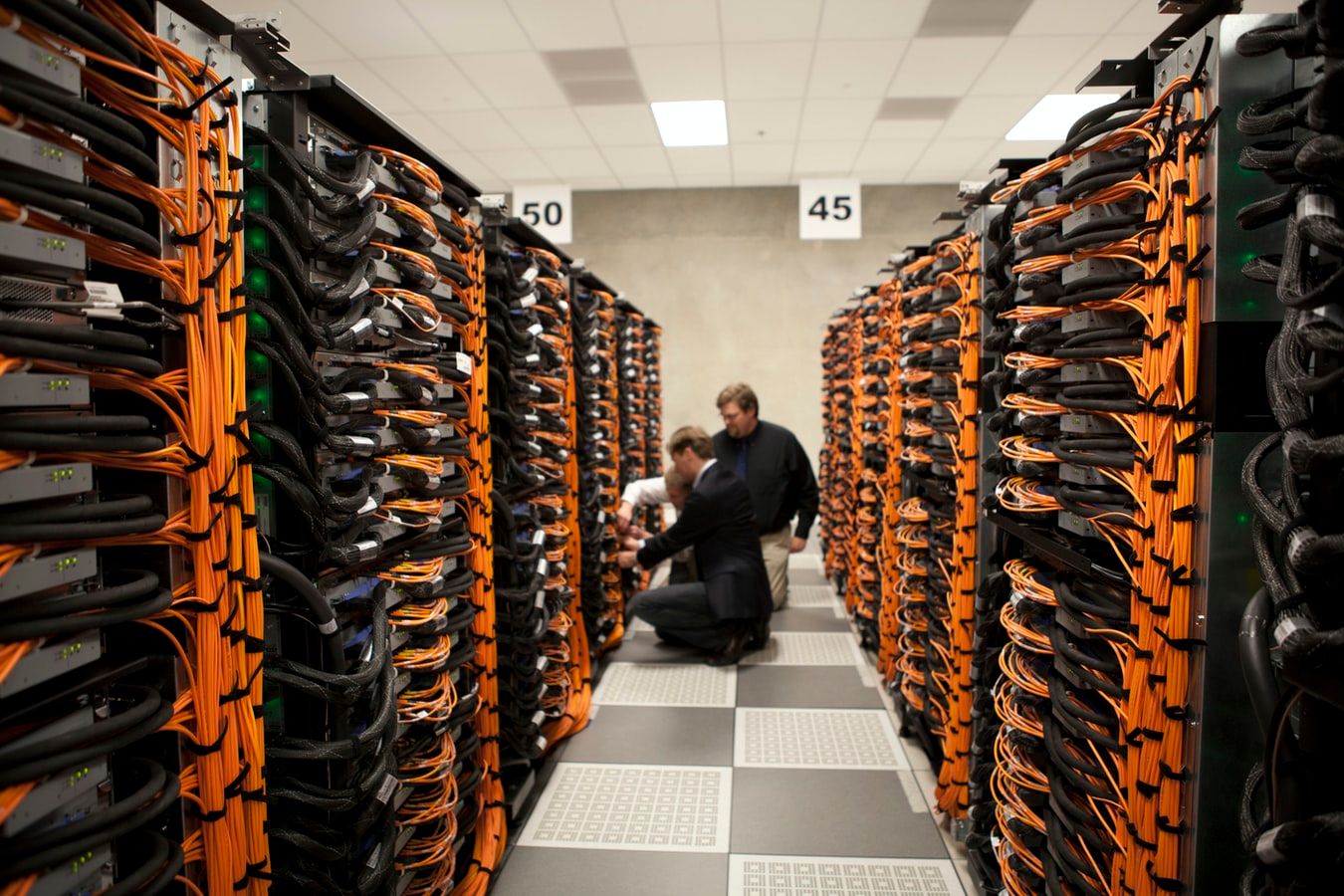